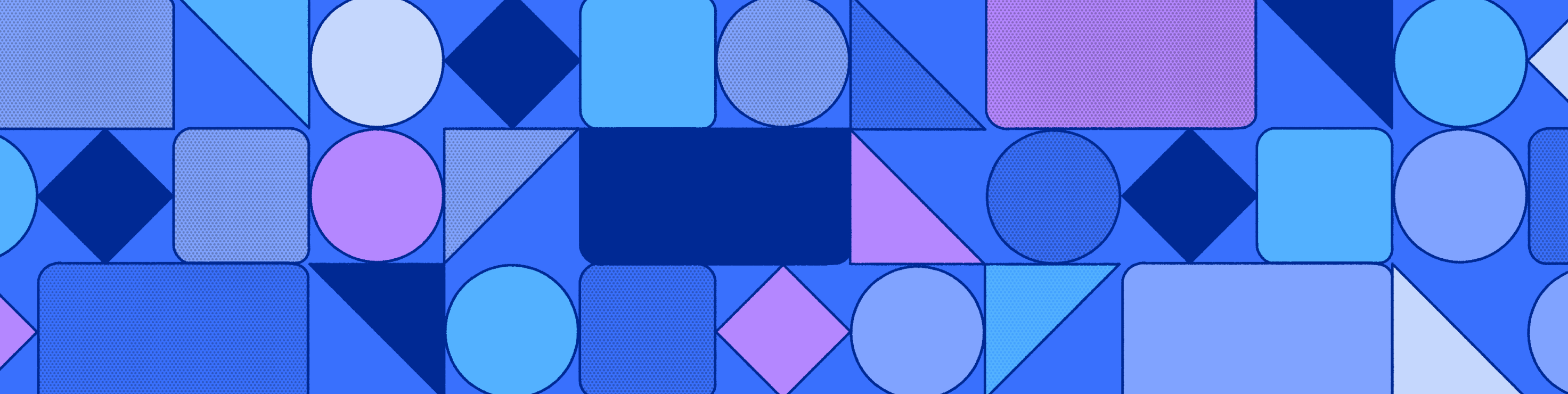
Combining Snowflake and PostgreSQL to build low-latency apps on historical data insights - APCJ
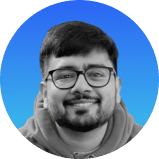

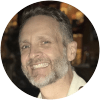
Snowflake’s new Hybrid Tables feature is designed to let customers build transactional apps directly on Snowflake. However, in some situations, users might prefer to sync Snowflake data to a dedicated transactional database like PostgreSQL for their low-latency workloads. In this webinar, we will show how you can surface historical insights from Snowflake via a transactional PostgreSQL layer to power real-time personalization in your apps. We will demo how Hasura and data APIs radically simplify this analytical + transactional architecture.
In our live eCommerce demo, we will use Snowflake as the customer system of record, with a batch ML model that continually calculates and updates the customer fraud risk score. We use Hasura to efficiently sync the select customer information to PostgreSQL as a speed layer. The fraud risk score in PostgreSQL is then processed via business logic to customize the user experience in real time. For e.g. show special promos for low-risk customers or disable credit eligibility for high-risk customers.
- Common use cases of “translytical” workloads
- Making intelligent real-time decisions based on insights from historical data
- Advantage of using data APIs to fetch information from a data warehouse
- Using Hasura to orchestrate the workflow
View Recording
Loading...
Snowflake’s new Hybrid Tables feature is designed to let customers build transactional apps directly on Snowflake. However, in some situations, users might prefer to sync Snowflake data to a dedicated transactional database like PostgreSQL for their low-latency workloads. In this webinar, we will show how you can surface historical insights from Snowflake via a transactional PostgreSQL layer to power real-time personalization in your apps. We will demo how Hasura and data APIs radically simplify this analytical + transactional architecture.
In our live eCommerce demo, we will use Snowflake as the customer system of record, with a batch ML model that continually calculates and updates the customer fraud risk score. We use Hasura to efficiently sync the select customer information to PostgreSQL as a speed layer. The fraud risk score in PostgreSQL is then processed via business logic to customize the user experience in real time. For e.g. show special promos for low-risk customers or disable credit eligibility for high-risk customers.
- Common use cases of “translytical” workloads
- Making intelligent real-time decisions based on insights from historical data
- Advantage of using data APIs to fetch information from a data warehouse
- Using Hasura to orchestrate the workflow